Introduction
Emotions are highly useful to model human behavior being at the core of what makes us human. Today, people abundantly express and share emotions through social media. Technological advancements in such platforms enable sharing opinions or expressing any specific emotions towards what others have shared, mainly in the form of textual data. This entails an interesting arena for analysis; as to whether there is a disconnect between the writer’s intended emotion and the reader’s perception of textual content.In this context we procure a Readers’ Emotion News datasets by using the social news network, Rappler and its award-winning Mood Meter widget. Mood Meter enables readers to cast their emotion votes towards several categories of emotions (Afraid, Amused, Angry, Annoyed, Don’t care, Happy, Inspired, and Sad) and records the total percentage of votes obtained for each emotion. Unlike other sources, we choose Rappler due to its simplicity, popularity, and ease of organizing several news articles under multiple genres and associated emotion profiles. We manually collect only the popular news articles by checking for high emotion votings represented in the Rappler Mood Meter, to ensure that the selected news articles have a high social reach. REN-10k is an advanced version of RENh-4k, in terms of the number of documents, length of documents, and much diverse set of emotion classes and document genres. This dataset contains 10,272 news documents with corresponding readers’ emotion profiles. Here, documents comprise news headlines, abstracts, and news content or fulllength news stories without non-textual content like images and videos. Unlike RENh-4k, readers’ emotion profiles are collected for a wider set of emotion classes: Afraid, Amused, Angry, Annoyed, Don’t care, Happy, Inspired, and Sad. We also assign documents to the categories Business, Entertainment, Lifestyle, Sports, Technology, and Others, by manually verifying genre information available in Rappler. REN-10k documents consist of the whole textual content associated with a particular news article, the average words per document is 533.613, i.e., long-text in nature. Since our study is over short-text documents, we utilize only the news headlines and associated abstracts of REN-10k to form the documents without the associated news content or full-length news stories.
Dataset Sample
News Headline: Countries ban China arrivals as virus death toll hits 213
News Abstract: Nearly 10,000 people have been infected in China by the new coronavirus and new cases are found abroad, with more than ...
News Content: BEIJING, China – Countries stepped up travel restrictions on arrivals from China on Friday, January 31, after a global health emergency was declared over a viral epidemic that has killed 213 people. Nearly 10,000 people have been infected in China by the new coronavirus and ...
News Category: Health & well-being
Readers' Emotion:
People
- Anoop K, University of Calicut, Kerala, India. (anoopk_dcs@uoc.ac.in)
- Deepak P, Queen’s University Belfast, Northern Ireland, UK. (deepaksp@acm.org)
- Savitha Sam Abraham , School of Science and Technology, Örebro University, Örebro, Sweden.
- Lajish V L, University of Calicut, Kerala, India.
- Manjary P Gangan, University of Calicut, Kerala, India.
Related Publication
Anoop K., Deepak P., Savitha Sam Abraham, Lajish V. L., Manjary P. Gangan. Readers’ affect: predicting and understanding readers’ emotions with deep learning. J Big Data June 2022, 9:82, Springer Nature, ISSN: 2196-1115, DOI: https://doi.org/10.1186/s40537-022-00614-2
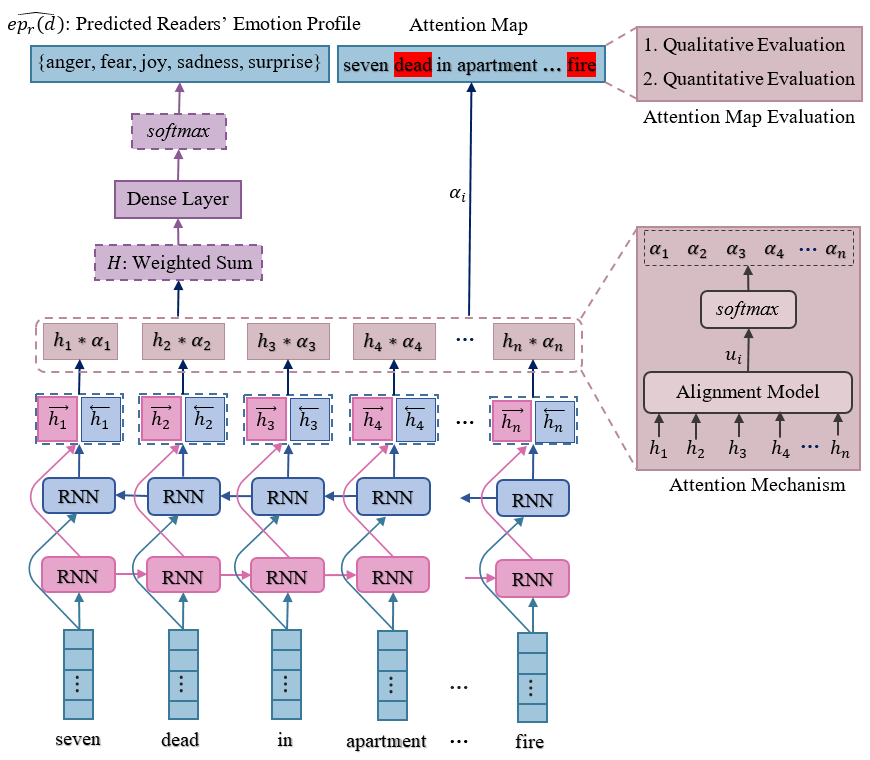
Abstract: Emotions are highly useful to model human behavior being at the core of what makes us human. Today, people abundantly express and share emotions through social media. Technological advancements in such platforms enable sharing opinions or expressing any specific emotions towards what others have shared, mainly in the form of textual data. This entails an interesting arena for analysis; as to whether there is a disconnect between the writer’s intended emotion and the reader’s perception of textual content. In this paper, we present experiments for Readers’ Emotion Detection through multi-target regression settings by exploring a Bi-LSTM-based Attention model, where our major intention is to analyze the interpretability and effectiveness of the deep learning model for the task. To conduct experiments, we procure two extensive datasets REN-10k and RENh-4k, apart from using a popular benchmark dataset from SemEval-2007. We perform a two-phase experimental evaluation, first being various coarse-grained and fine-grained evaluations of our model performance in comparison with several baselines belonging to different categories of emotion detection, viz., deep learning, lexicon based, and classical machine learning. Secondly, we evaluate model behavior towards readers’ emotion detection assessing attention maps generated by the model through devising a novel set of qualitative and quantitative metrics. The first phase of experiments shows that our Bi-LSTM+Attention model significantly outperforms all baselines. The second analysis reveals that emotions may be correlated to specific words as well as named entities.